Ask Me Anything: Dynamic Memory Networks for Natural Language Processing
Goal
a framework for general question answering tasks trained using (input, question, answer).
Tasks
sequence tagging, classification, sequence-to-sequence(seq2seq), question answering(QA) that requires transiitve reasoning.
Model
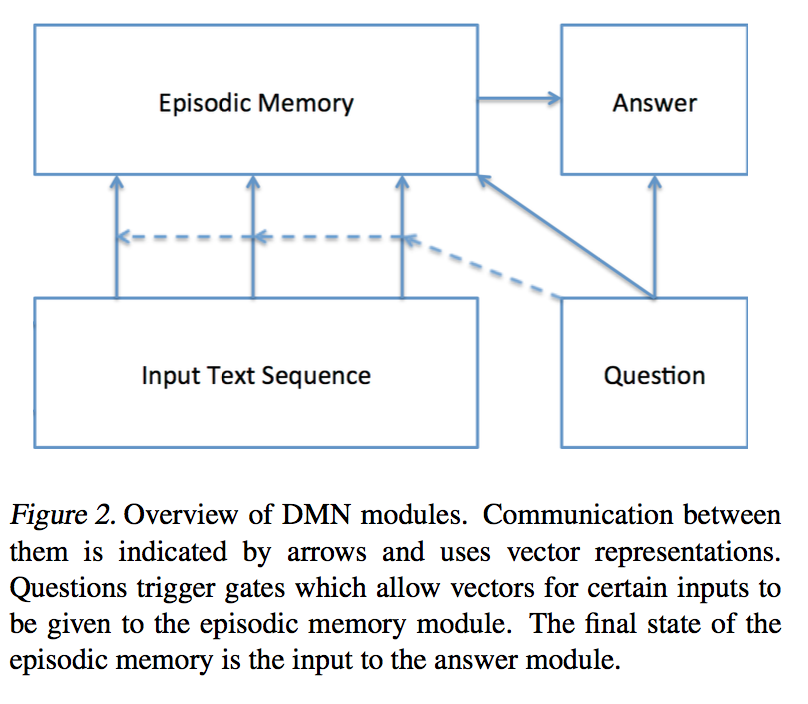
encode raw inputs(images, texts(sentences, story, tweets…etc) ) to distibuted vector representation.
GRU is used instead of LSTM for computation efficiency with similar performence.
Question Module
encode raw question of tasks. Then fed into espsodic memory module(EMM) as initial state/basis for it(EMM) to iterates.
Share word embedding matrix accross Input module and Question Module.
Episodic Memory Module
Iteratively attend different part of the input** given the current state.
(share some similarity to hops between controller module and memory module from End-To-End Memory Networks(MemN2N))
Compromised of an attention mechanism within a recurrent network.
Score function G of the answer is an 2-layer feed forward Neural Network as well.
Criteria for stopping includes a end-of-passes representation for the GRU to choose as well as a maximum number of iteration just in case.
Answer Module
Generate answer from final memory vector from EMM.
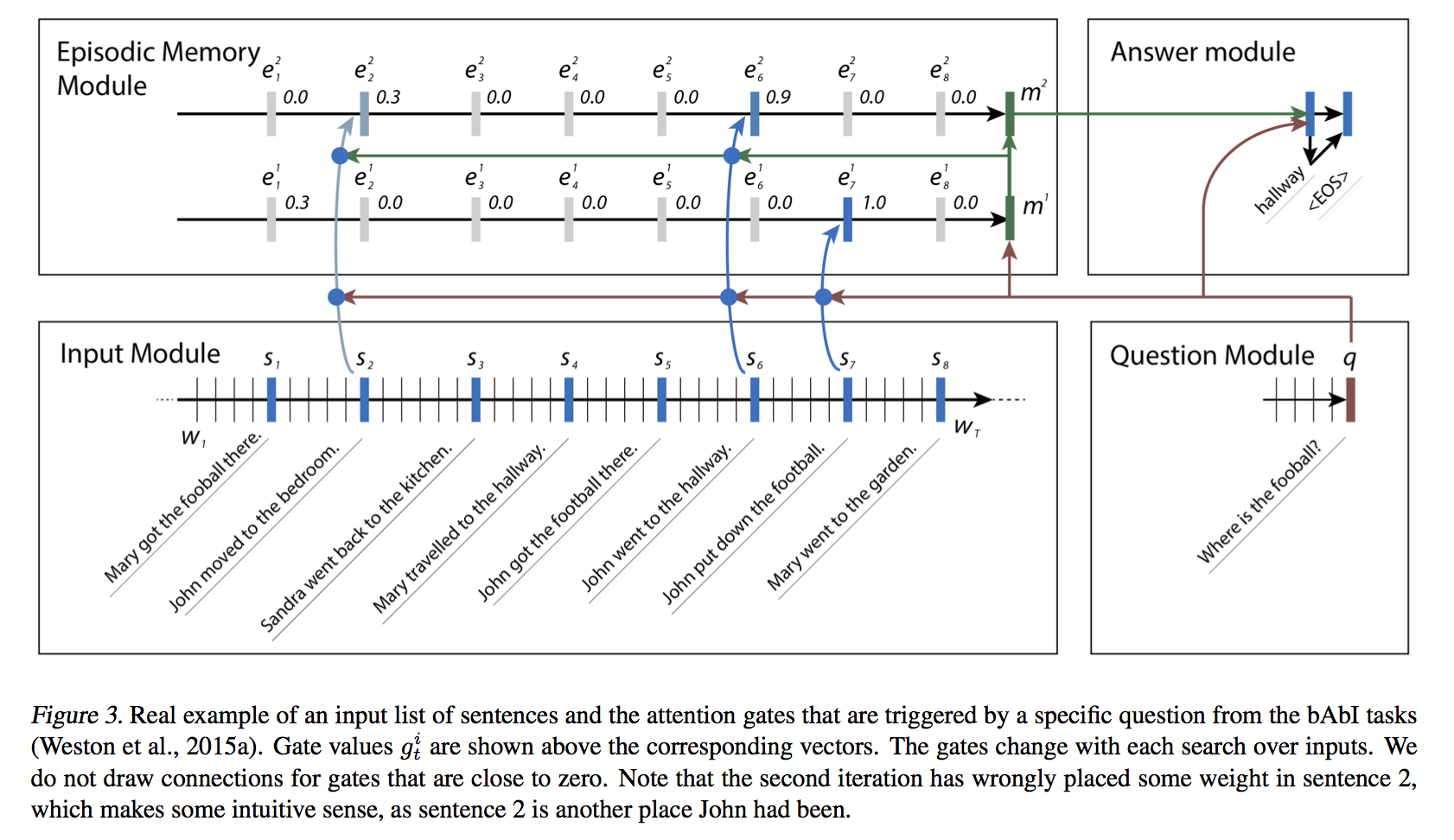
Training
Experiments
###QA
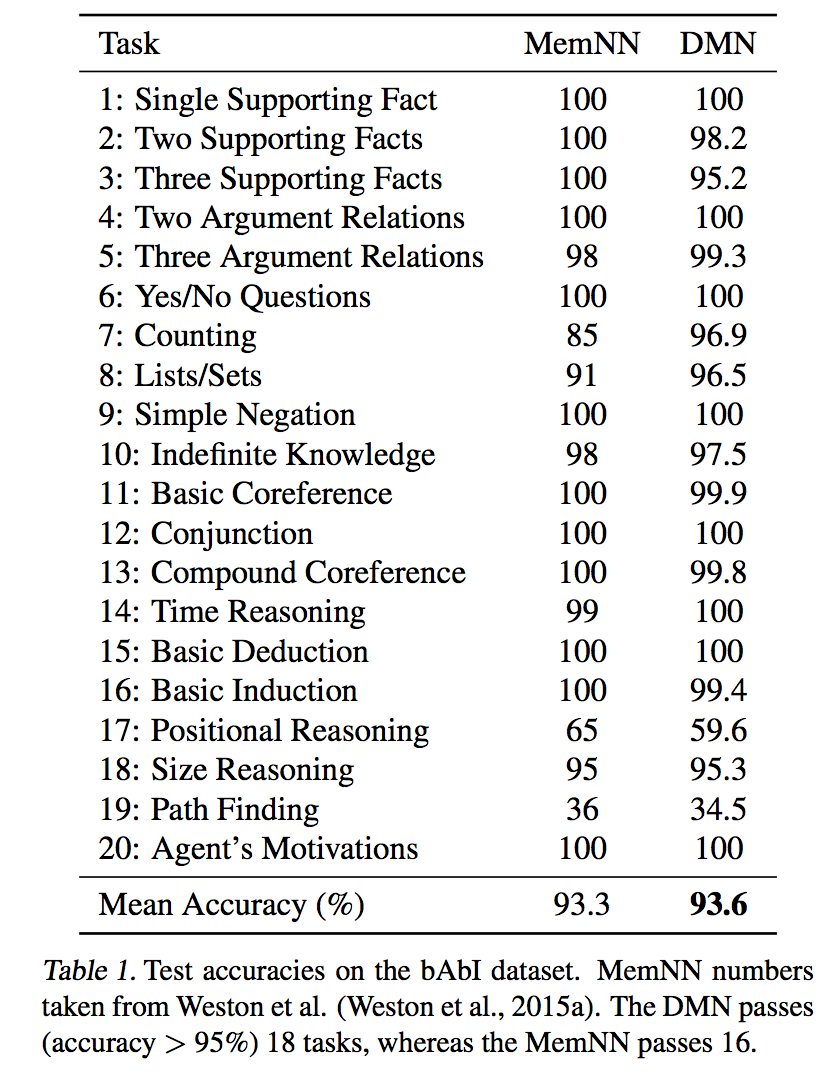
TODO: Experiments is not complete